Control Spamming
Control Spamming
Spam Detection is a monitored machine learning challenge. Spam Detection isn’t a new problem yet it remains colossal to date. How do we identify spam using NLP techniques? This write-up will shed light upon the interpretation and analysis of texts by computer systems and how to filter out spam using NLP and other machine learning models.
Information extraction helps obtain structured information from unstructured or semi-structured documents like social media posts & more
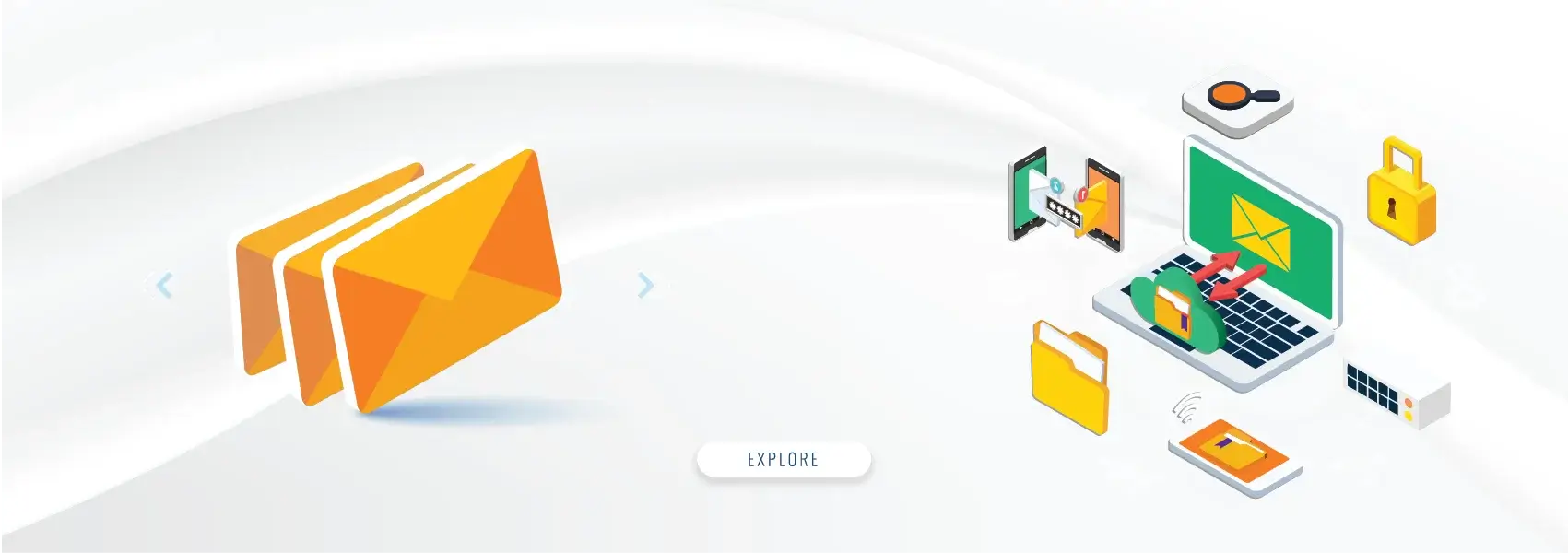
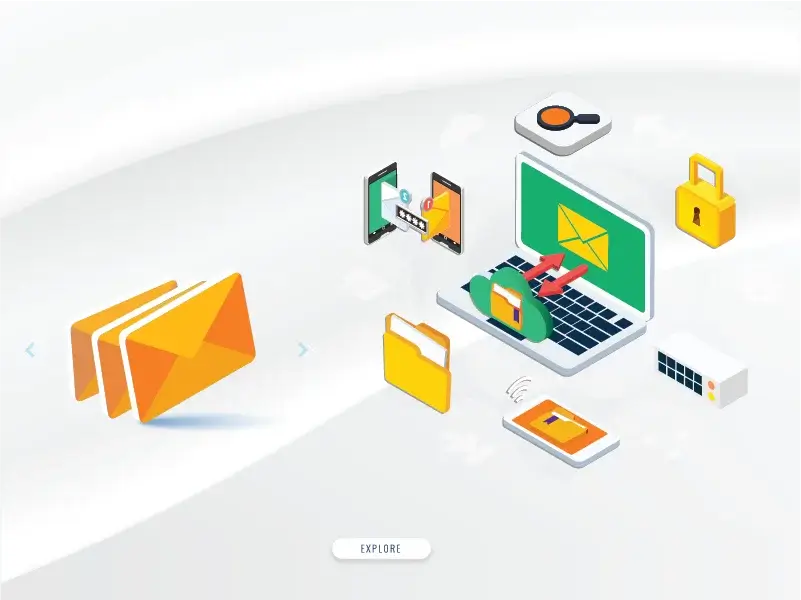
Control Spamming
Spam Detection is a monitored machine learning challenge. Spam Detection isn’t a new problem yet it remains colossal to date. How do we identify spam using NLP techniques? This write-up will shed light upon the interpretation and analysis of texts by computer systems and how to filter out spam using NLP and other machine learning models.
Information extraction helps obtain structured information from unstructured or semi-structured documents like social media posts & more
Spam Detection as a Challenge
Spam comes in various colors. Some are just troll messages aiming to have attention for any possible motive, some are just pishing links planning to infiltrate your web, and some are just there to spread false information. What all these have in common is that they are irrelevant and meaningless to the recipient.
A spam-detector algorithm is used to filter out spam and avoid flagging authentic messages. It must be done in a way that evolving trendy searches don’t get detected as spam such as election news, cryptocurrencies, and the pandemic, which is why Spam Control becomes a tricky chore to get through.
Naïve Bayes machine learning and spam detection
For the classification of data, statistical models are used by machine learning algorithms. Any trained machine learning model should detect spam but the one with the widest appeal is the “naïve Bayes” algorithm. It’s called “naïve” as it assumes features of observations are not dependent. In the case of spam detection, the features are word combinations or words in the email’s text.
Spam Detection using NLP
Without providing an example of ham and spam messages, you cannot strengthen your machine learning algorithm to separate relevant ones from irrelevant ones in Natural Language Process (NLP). Many email providers have their labeled emails for the purpose and using that each company has its mechanisms for the “Report Spam” feature. Besides, lemmatization and stemming are also the right techniques for simplifying your machine learning model.
Spam detection NLP is always in progress as there is no certain limit or stopping of spam content. Even though developers use AI, some of those messages get their junk past the filters. That’s exactly why NLP Australia resorts to further human help to update and improve their spam detectors.